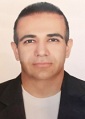
Ali Shaeri
The National Iranian Oil Engineering & Construction Co., Iran
Title: Optimization of delay coker unit by using of artificial neural network
Biography
Biography: Ali Shaeri
Abstract
A Feed forward and multilayer Perceptron Artificial Neural Network (ANN) with back propagation algorithm was applied for optimization of Delayed Coking Unit (DCU). To this aim, one year of operating conditions were gathered from Distributed Control System (DCS) and laboratory data. Then all data were evaluated by experienced operators to determine the most effective operating condition. Conradson Carbon Residue (CCR), asphaltenes content and Feed API were selected for input of the ANN and the output was set to be maximum products yield. The inputs of neural network data are distributed for training, validation and testing sets in 60%, 20% and 20% ratios, respectively. A three-layer ANN was adopted to predict products yield in terms of aforementioned inputs. To find the best fitted ANN structure, several structures (around 1576) were examined. Here, a total of four combinations of transfer functions were tested against various training functions available in the MATLAB software. Only the random order incremental training with learning functions (TRAINR) and the Levenberg–Marquardt (TRAINLM) training functions were found to predict significantly accurate results. It was found out that the best structure had 4, 6 and 1 neuron for the first, second and third layer, respectively. Furthermore, logsig, logsig and purelin were found the best transfer function for first, second and third layer. The best model was extracted and the obtained data were applied to the live DCU with the capacity of 90000 bbl d-1. Transfer functions are generally assigned to a network layer to first process the input signal, followed by the calculation of suitable weight for the output signal such that the relations of the data set can be identified. According to many structures which can be used in ANN, an optimization method using correlation coefficient (R) was recommended to optimize the number of neurons and transfer function types. The results showed that the selected model could be exactly matched with the DCU.